In the age of artificial intelligence (AI), data is the lifeblood that fuels innovation, drives insights, and powers intelligent decision-making. However, the exorbitant volume, variety, and velocity of data can pose significant challenges without a well-defined data management strategy in place. In this article, We’ll delve into the essential steps to create a robust data management strategy tailored specifically for AI initiatives.
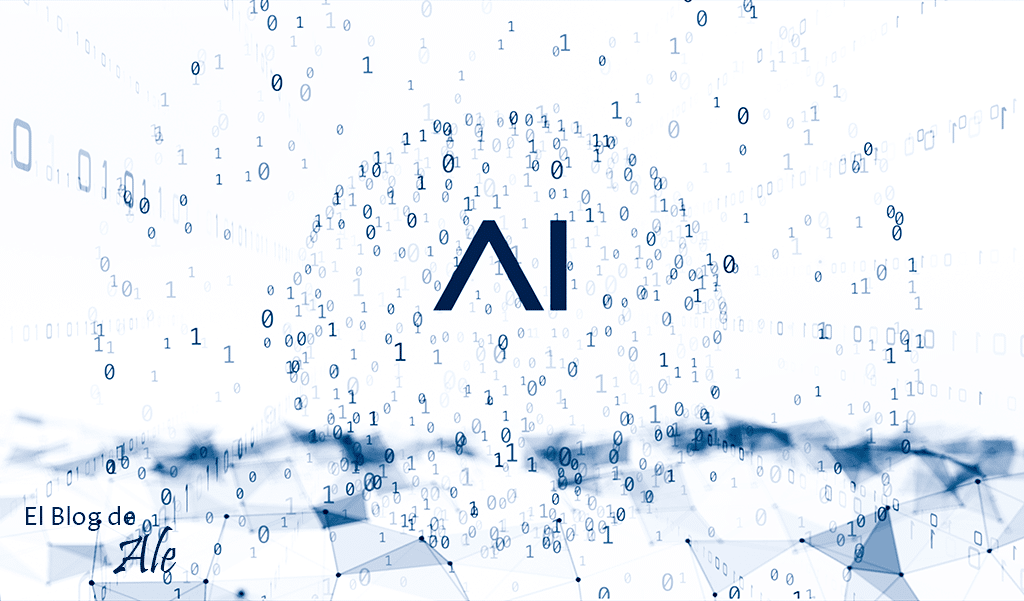
Define Objectives and Goals
Before embarking on designing a data management strategy, it’s crucial to clearly outline the objectives and goals of your AI projects. Whether it’s enhancing customer experiences, optimizing operations, or developing predictive models, understanding the end goals will shape your data management approach.
You might be interested: Setting Clear Objectives and Goals for Data Management Projects
Establish Data Governance
Data governance forms the foundation of effective data management. Define policies, processes, and procedures to ensure data quality, security, privacy, and compliance with regulatory requirements. Assign roles and responsibilities for data stewardship and oversight to maintain accountability and transparency.
Inventory and Catalog Data Assets
Create an inventory of all available data sources within your organization. Catalog metadata such as data formats, structures, geographical locations and relationships to facilitate data discovery and understanding. This step is essential for identifying relevant data assets and determining their suitability for AI applications.
Ensure Data Quality and Integrity
Data quality is so important for the success of AI initiatives. Implement processes for data profiling, cleansing, and validation to identify and rectify inconsistencies, inaccuracies, and missing values. Continuous monitoring and improvement are key to maintaining data quality over time.
Implement Data Security Measures
Protecting sensitive data from unauthorized access, breaches, and misuse is critical. Implement robust security measures, including access controls, encryption, and data masking, to safeguard data throughout its lifecycle. Ensure compliance with data protection regulations such as GDPR, CCPA, and HIPAA.
Integrate and Consolidate Data Silos
Break down data silos by integrating disparate data sources to create a unified view of your data landscape. Utilize modern data integration tools and platforms to streamline the process of extracting, transforming, and loading (ETL) data from diverse sources into a centralized repository.
The use of Data Replications solutions based in Change Data Capture technology could be critical to keep data always in synch.
Optimize Data Storage and Infrastructure
Select scalable and cost-effective data storage solutions that meet the performance and scalability requirements of AI workloads. Cloud-based storage options such as Amazon S3, Google Cloud Storage, or Azure Blob Storage offer flexibility, scalability, and reliability for managing large volumes of data.
Establish Metadata Management Practices
Implement metadata management systems to catalog, track, and manage metadata associated with your data assets. Maintain comprehensive metadata repositories that capture information about data lineage, provenance, quality, and usage to enhance data governance and decision-making.
Define Data Lifecycle Management Policies
Define clear policies and procedures for managing the lifecycle of data from creation to archival or deletion. Establish data retention schedules, archival processes, and disposal guidelines to optimize storage resources and ensure compliance with legal and regulatory requirements.
Foster Collaboration and Knowledge Sharing
Promote continue collaboration and knowledge sharing among data stakeholders, including data scientists, analysts, engineers, and business users. Facilitate open communication and collaboration to leverage collective expertise and insights for driving AI innovation and business value.
Monitor, Measure, and Iterate
Implement monitoring and measurement mechanisms to track key performance indicators (KPIs) related to data management, such as data quality, security incidents, and compliance adherence. Continuously monitor, analyze, and iterate on your data management strategy to adapt to changing business needs and technological advancements.
Conclusion
A well-crafted data management strategy is essential for unlocking the full potential of AI and driving business success. By following the outlined steps and best practices, organizations can establish a solid foundation for managing data effectively, ensuring its quality, security, and accessibility for AI-driven insights and decision-making. Embrace the power of data management to fuel innovation, drive competitive advantage, and realize the transformative potential of AI in your organization.
3 thoughts on “Be Ready for the Future: How to Prepare a Data Management Strategy for Artificial Intelligence”
Comments are closed.